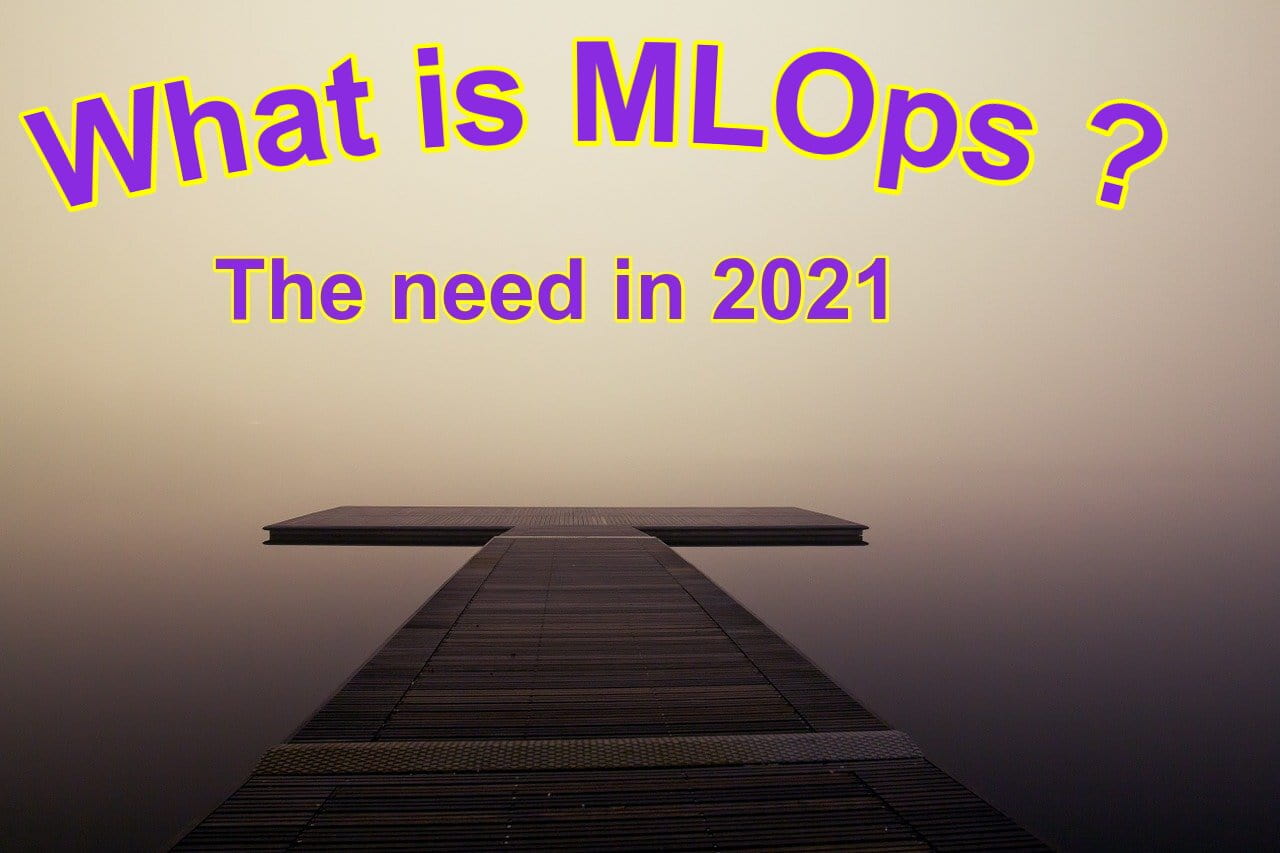
MLOps: When DevOps meets Data Science
Table of Contents
MLOps – When Data Scientists use DevOps
MLOps stands for Machine Learning Operations. It’s basic communication between Data scientists & the Operations or Production team. It is designed to eliminate waste, automate as much as possible & produce richer, consistent insights with machine learning.
MLOps includes a set of best practices for organizations to run AI successfully with help of software products and cloud services.It uses Data Science and Operations teams to improve model deployment through Machine Learning (ML), AI, monitoring, collaboration, and communication.
MLOps is quite a new field because the commercial use of AI itself is fairly new. Most data scientists are not hard-core programmers. They can create a great model for a machine learning problem. But, they don’t have the skills to package, test, deploy & maintain this model in production.
That’s where MLOps come to use. MLOps goes above and beyond the model development and design. By bringing DevOps principles to machine learning, it enables a faster development cycle & better quality control.
Why MLOps?
MLOps or DevOps for machine learning is bringing the best practices of software development to data science. It allows data scientists the freedom to do what they do best- find answers, integrate machine learning into your software development division.
This way you can create reactive development processes that will unite teams across your business. Because you hired a data team for their skills in information gleaning. MLOps follows a similar pattern to DevOps. These practices drive a seamless integration between the development cycle & overall operations cycle.
MLOps is becoming an essential skill-set. It concentrates on enterprises looking at the benefits of machine learning in the real world. It’s a practice for collaboration and communication between data scientists and engineers to improve automation. Microsoft Azure Machine Learning offers enterprise level capabilities to accelerate the machine learning lifecycle. When you adopt an MLOps approach, it will help your organization in the following ways:
- MLOps combines the expertise of operations and data scientists. The operation team has the business knowledge and your data science team understands the data ML leverages both skillsets.
- MLOps puts the operation team at the forefront of new regulations and best practices. They can take ownership of regulatory processes and the data team can concentrate on creative model deployment.
- Results from complicated & non-intuitive algorithms ease with a better division of expertise along with a bigger collaboration from operations and data teams. MLOps tightens the loop.
MLOps Roles & Responsibilities
MLOps helps increase automation & also improves the quality of production ML while focusing on business & regulatory requirements.
There are 3 key components/roles of MLOps:
- Machine learning
- DevOps (IT)
- Data Engineering
Each role contributes to key elements that work closely with the ML lifecycle loop. It’s a good idea to consider a few things before building MLOps into your application. Your organization needs to settle a few things before you bring ML into production.
1. Know your benchmarks:
KPI should be clear and measurable so everyone is on board. Data science experts understand what is at stake and the operation team knows how to use insights to move forward.
2. Who is monitoring?
ML uses non-intuitive mathematical functions. It requires constant monitoring to ensure you’re operating within regulation. You have to retrain data periodically & determine how and when to do so. It requires critical collaboration between the teams involved.
3. How MLOps works?
MLOps follows a similar pattern to DevOps. MLOps is the process of taking both data & code to produce predictions that describe which deployment to put into production. It requires code as well as data teams to work together.
Benefits of MLOps
- MLOpsoffer rapid innovation through robust machine learning lifecycle management.
- It will do effective management of the entire machine learning lifecycle.
- It deploys high precision models quickly in any location.
- MLOps inherits the key DevOps concepts such as automated validations, testing, profiling, and environment management.
- MLOps creates reproducible workflows and models.
- It keeps track of version history and model origin to enable auditing.
- It helps to manage and maintain the partnership of data science and IT/Ops teams to work in collaboration and provide ML-powered applications.
Limitations of MLOPs
- Monitoring and management
- Business uses
- Governance and regulatory compliance
- Deployment and automation
- Reproducibility of models and predictions
- Diagnosis
- Scalability
- Collaboration
The above limitations are as described in Wikipedia. However, with careful consideration and knowledge of the difficulties, you can reach your MLOps goal smoothly by implementing standard practices. Some of the standard practices are ML pipelines, co-team operations, monitoring, validation, and versioning.
The ML gives importance to the need for teams that can autonomously develop and deploy ‘production level’ ML Solutions. The capabilities of MLOps are essential to support data science use cases. To execute a successful data science strategy, it is crucial to implement MLOps consistently.
The future of MLOps
As the data science industry starts focusing on scalability, greater collaboration, and the need for machine learning models to be production-ready, MLOps is becoming a vital tool and process.
So as we gear ourselves for moving into the next decade, MLOps definitely is going to be a technology that is for sure going to take the world by storm.With increasing use of data science and AI based technologies, MLOps will play an important and imperative role in the growth of all domains and industries.